This is the third article in a series of blog posts in which we introduce the concepts of knowledge-based lending for SME business. In this article, we look at the opportunities and challenges provided through rating and scoring methodologies. We provide a general overview of those tools to support lending and monitoring. We show the limitations with regards to automated decision support.
Rating and scoring methodologies are integrated into Q-Lana, the digitization platform for knowledge-based lending. We have developed Q-Lana as a comprehensive, fully customizable and intuitive, filled with smart tools and analytics to support financial institutions. For more information about Q-Lana, please read below and contact us.
The availability of technology has created a wave of initiatives to simplify the lending process through scoring and rating methodologies[*]. The expectations related to rating and scoring methodologies are high, if not exaggerated. In this article, we explain the various methodologies in simple terms, without getting too mathematical. We show how they can support financial institutions in an intelligent way in the decision making and monitoring of loan exposures to corporates and SMEs.
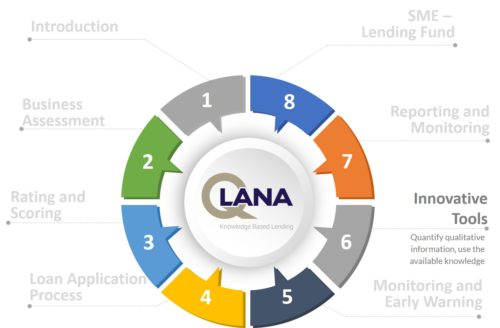
Rating and Scoring in simple Terms
Rating and scoring models aggregate information about credit risk into a single number, a score. This score shall classify the exposure, ideally by associating a default probability. Combined with the estimated loss in the case of default and other input factors, the total expected loss of a loan exposure can be calculated. Regulators appreciate the efforts made by financial institutions to analyze the risk in a structured way. This allows a better alignment of the regulatory capital requirements with the actual economic capital needs based on the risk profile.
Three types of information are used for the assessment of a rating:
- Quantitative data about the borrower, objectively measurable numerical values, derived from primarily financial information
- Qualitative data, derived from assessment and monitoring of the borrower, the business strategy, management qualification and other relevant factors
- External information about prices and economic indicators which are deemed relevant for the performance of the borrowers
Types of Rating Models
The rating and scoring models, used by financial institutions can be split into three main categories:
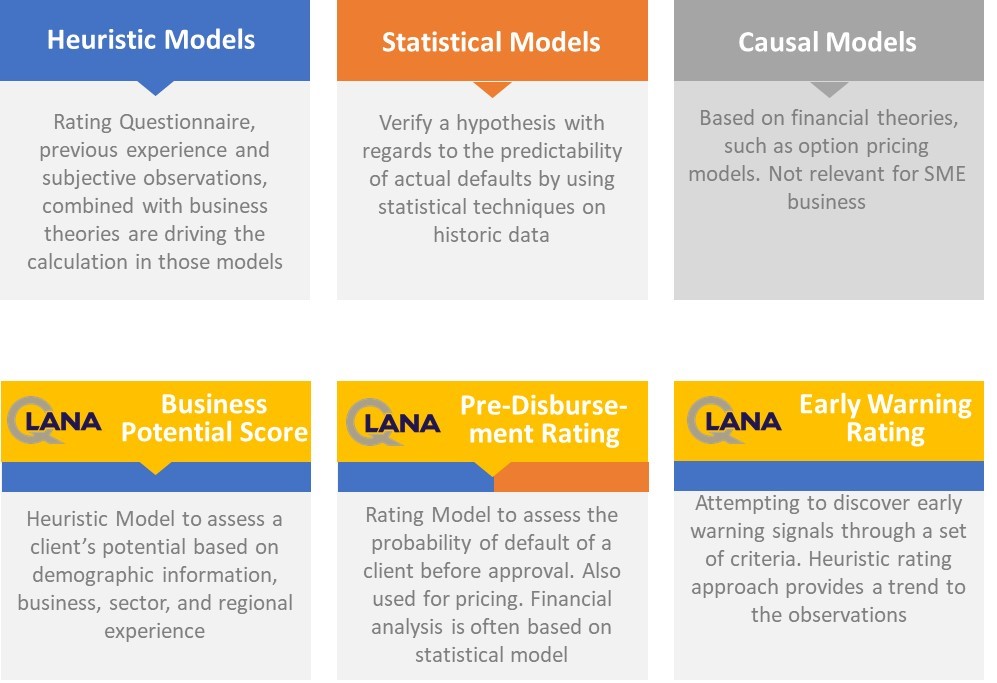
- Heuristic models – previous experience and subjective observations, combined with business theories are driving the calculation in those models. There is usually no statistical validation or optimization. The heuristic models are frequently in the form of rating questionnaires which are built by credit experts. Ideally those questionnaires have clearly defined answers, leaving little discretion to the users. The quality of the models depends on the experience of the developers and users as there will always be judgement involved in the answers. The actual grading associated with the selected answers is subject to the presumed impact on the credit quality. Qualitative assessments are used, in which specific criteria are assessed and classified for example as “good”, “average”, or “poor”, based on comparative analysis and judgement. The usefulness of heuristic rating tools depends largely on the detail of instructions and the discipline of the users in assessing borrowers. The result of a heuristic rating is a score, which can be categorized into a rating classes (e.g. AAA, AA, A, ..). The number of rating categories can be freely assigned. Regulators have certain minimum requirements to accept the rating as an approved internal rating model. The heuristic models are the basis of the borrower/obligor ratings of most of the established rating agencies. Through back testing the rating models over longer periods of time, rating agencies fill migration tables which allow to derive the default frequencies for rating classes. Yet, established rating agencies tend to shy away from making a strong connection between those factors in the actual rating process.
- Statistical models – the statistical models attempt to verify a hypothesis with regards to the predictability of actual defaults by using statistical techniques on historic data. The quality of the statistical tool depends very much on the empirical data used in the model development. This requires a high quality data set about a large number of borrowers. There also needs to be a sufficiently large number of actual defaulted loans, to calibrate the model. Data quality is another key criterion to ensure that the statistical model produces meaningful results. The statistical instruments used to calibrate a statistical model range from regression analysis, discriminant analysis, profit analysis, and logistic regression to more advanced methods, such as linear programming, decision trees, and neural networks. The criteria used in statistical models include for example demographic data (age, gender, nationality), data derived from past lending activities (credit bureau information, past arrears data) as well as more recently also psychometric data such as the time it takes to answer certain questions.
- Causal Models are the third category of rating models. They are based on financial theories, such as option pricing models. It is assumed that a credit default will occur when the economic value of the borrower’s assets falls below the economic value of its debt. Pre-condition for such models is the availability of market prices for debt and equity, which makes the model difficult to pursue for most borrowers.
In real world applications, the methodologies are often combined. Most common are rating models which use a combination of heuristic and statistical data.
Issues with Rating and Scoring
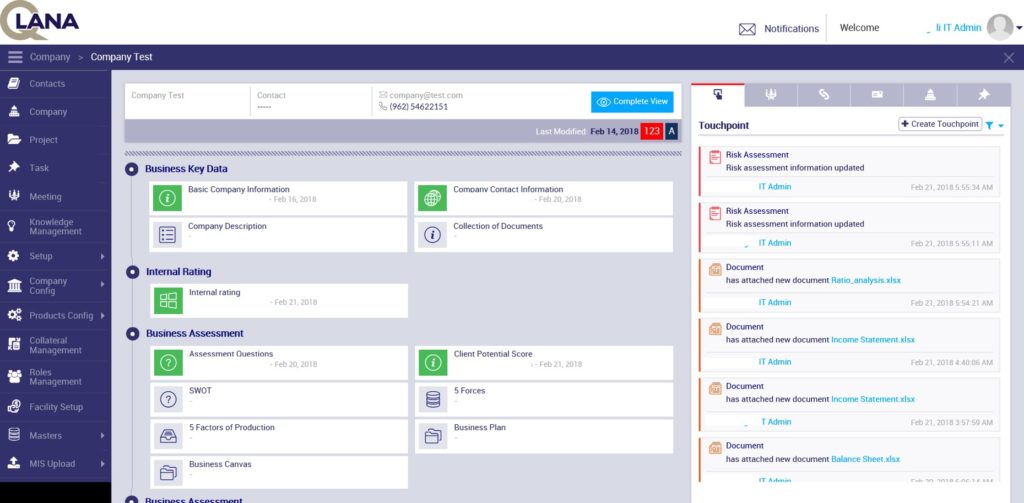
In our work with financial institutions to develop and implement rating and scoring models, we often came across the same issues related to the availability and quality of data:
- The financial institutions have not conducted a sufficiently large number of loan approvals to properly calibrate a statistical scoring model. While there is no minimum number of data sets, it is recommended to have at least 5000 or more homogeneous loans to validate a statistical model. The idea to use data from other institutions or potentially from purchased databases is usually not compensating to the lack of own information
- There also needs to be a sufficiently large number of defaults (>5%) for the right calibration. In practical applications, we often have to redefine the default criteria, in order to come up with a sufficiently large number of “bad cases”.
- Data quality is challenging in most of the financial institutions. Demographic data is usually overwritten by the accounting system: when downloading the information of a borrower who took 10 loans from one financial institution over the last decade, the demographic data associated with this loan reflects only the latest information but not the information from previous loans. For example, the borrower’s marital status might currently be “widowed”. When downloading the information of the past loans from the accounting system, the marital status is the same for all the loans, as it is derived from the database of key client data. The borrower might have been “single” for the first loans, “married” for several later loans and has only been a “widow” in the last loan cycle.
- Financial institutions use open text fields for the collection of data, instead of drop downs. As a result, the number of different values for certain categories is exaggerated. When information is collected in free text format, similar values are expressed differently. For example, it might be a difference whether the client is based in the “Western region”, “West region”, or simply “West”. There is a difference in descriptions, whether the client is classified as “Head of Marketing”, or the “Marketing Head”. Those quality issues are on top of the other expected problems, such as available data, wrong data, or data classified as “other”.
- Financial institutions with a “bricks and mortar” business model have a largely implicit natural pre-selection of borrowers. A loan officer will not prepare an application for every potential borrower and will only present cases to the credit committee which have a chance of approval. Such “pre-declined” low quality borrowers will not be captured in the systems. In addition, traditional accounting systems do not capture transactions which are declined or which are not completed by the borrower.
Those issues with regards to data quality and data availability make it difficult for financial institutions to develop statistical scoring models.
When developing Q-Lana, we looked at the most pragmatic ways of applying rating and scoring methodologies. Q-Lana as an integrated rating widget, which can be deployed in several instances in the lending process. The widget is set up to function as a heuristic rating tool, with the potential to include statistical rating components. We suggest using the rating tool for the following purposes in a best practice lending model:
- Assessment of the client’s business potential. Over the recent years, studies have been conducted to assess which companies have the best preconditions to become attractive clients for full-service financial institutions in the SME sector. For example, a study conducted by Center for Financial Inclusion lists the following criteria as important for small enterprises to grow successfully (Emerging SMEs – Secrets to growth from micro to small enterprise, October 2016, Christy Stickney): age of the borrower (ideally between 30 and 49 years old), exclusive dedication to operating a single business, business development based on experience and vision, maintenance of written financial accounts and several other criteria. The rating widget of Q-Lana can be used in the initial client assessment process, or to derive the business potential of a company. This can help the financial institution to offer specific targeted financial services to this company.
- Assessment of the credit quality for the loan approval. This is the most common application of the rating model. The Q-Lana rating widget allows the financial institution to define heuristic and statistical rating criteria flexibly for several borrower types in a variety of sectors. The rating widget is fully customizable with regards to the specific criteria, the quantification of those criteria and the weighting of the criteria against each other. A rating which is calculated in the context of a loan application can be used for decision support as well as for pricing and approval limits. From our perspective, more important than the absolute rating value is the comparison of borrowers against each other.
- Early Warning Rating. Q-Lana suggests adjusting the rating for the monitoring of existing transactions. As will be explained in a later chapter of this blog, high importance is given to the monitoring of existing exposures. A specific early warning rating has been prepared for the users of Q-Lana. This rating considers performance information of a borrower under an existing loan exposure and monitors trends in the development of credit quality. Such infoArmation is usually not available for clients during the application process. More important than the absolute value of the rating is the trend of the rating development of an individual borrower.
Q-Lana provides the rating tools in a flexible and fully customizable way. When customizing Q-Lana for a financial institution, we provide guidance on the customization of the tools based on our experience and best practice. The tools can all be updated over time as more information is collected and better assessments can be made.
We see this usage of the rating tools as the best way to take advantage of the technology, without overstretching the concept. In the next article of the blog, we will describe the process of loan application in which we added additional innovative tools and instruments for a better risk assessment.
About the author:
Christian Ruehmer is the Co-Founder and CEO of Q-Lana. Christian has over 25 years of experience in finance working for several large international banks in the areas or Risk Management, Credit Portfolio Management, and Investment Management. Christian has been an advisor in this sector, working with over 75 MFIs, banks, and international organizations, primarily in developing countries. He is the head of Risk and Compliance at Bamboo Capital Partners and sits on the board of several companies in the finance sector
About Q-Lana:
Q-Lana provides a digital platform to transform traditional lending into a knowledge-based, risk focused process. By digitizing the initial assessment, application and approval, monitoring and portfolio management process, as well as the collection, the financial institution generates significant knowledge about the performance and the credit quality of the target clients. This can be used to improve lending decisions, avoid losses and provide advanced support to the clients. Q-Lana replicates the existing lending process and allows for modifications to apply best practice. In addition, Q-Lana provides intelligent reporting and customized risk advisory. Q-Lana is based on a SaaS model that integrates well with existing core banking software and can be implemented within a 3-4 month timeframe.
Contact:
christian@qlanaq-lana.com
[*] We use the expression “rating model” in the context of credit assessment of a borrower and “scoring” as a component of a rating model. In the industry, there is no clean separation in the use of the expressions